R Biplot Psych
Added a biplot.psych function to do biplots of fa or principal results. Added an unbiased estimate of kurtosis in the kurtosi function. Added a predict function to fa and principal (predict.fa and predict.principal, both called from predict.psych) Added lavaan.diagram to draw the output from a cfa or sem from the lavaan package. Scale # covariance biplot (scale = 1), form biplot (scale = 0). When scale = 1, the inner product between the variables approximates the covariance and the distance between the points approximates the Mahalanobis distance. Obs.scale # 标准化观测值 var.scale # 标准化变异 pc.biplot # 兼容 biplot.princomp groups # 组信息. Rotations and transformations are either part of psych (Promax and cluster), of base R (varimax), or of GPArotation (simplimax, quartimax, oblimin). Some of the statistics reported are more appropriate for (maximum likelihood) factor analysis rather than principal components analysis, and are reported to allow comparisons with these other models. R/biplot.psych.R In psych: Procedures for Psychological, Psychometric, and Personality Research Defines functions plotone. #rewritten Sept 18,2013 to.
Working on a recent project to characterize the underlying factors of urban typology, we needed a quick means of visualizing the factor loadings of each indicator. We had used the “pysch” library’s “fa” function to get the analysis done. However, a quick visualization approach was not forthcoming. One of my research assistants found this fantastic example using ‘ggplot2’. However, getting the ggplot function call to work was not quite as straightforward as in the example shown.
In case you are in a similar situation, where you have obtained the loading matrix directly from the ‘fa’ function and want to use the beautiful rendering of the ggplot facet-bar approach, below is code that you might find useful.
The additional steps involve converting the loading matrix to a dataframe and then converting the row names to a dataframe column. Ignore the descriptive factor names chosen for this example, and the input file can be dataframe or matrix.
2 4 6 8 10 12 14 16 18 20 22 24 26 28 30 32 | library(data.table) library(reshape2) options(width=240) df=read.csv('precities.csv') #scree(df) #fa.parallel(df2) #loadings # print(fa(df,9,rotate='oblimin',fm='minres')$loadings,cut=.2) load=load$loadings load=data.frame(load) colnames(load)[1]<-'Indicators' colnames(load)[2:10]<-c('Urbanization','Density, metro','Cost, innovation','Public/active transit','Congestion','BRT','Bikeshare','Urban poverty','Urban sprawl') load.m<-melt(load,id='Indicators',variable.name='Factors',value.name='Loading',measure=colnames(load)[2:10]) #load.m <- melt(load, variable.name='Factors', value.name='Loading', measure = c('Urbanization','Density, metro','Cost, innovation','Public/active transit','Congestion','BRT','Bikeshare','Urban poverty','Urban sprawl')) loadPlot<-ggplot(load.m,aes(Indicators,abs(Loading),fill=Loading))+facet_wrap(~Factors,nrow=1)+geom_bar(stat='identity')+coord_flip()+ scale_fill_gradient2(name='Loading',high='blue',mid='white',low='red',midpoint=0,guide=F)+ylab('Loading')+theme_bw(base_size=10) |
Hi,
I have a prcomp object (generated using the prcomp function) and I am trying to generate a biplot using ggbiplot, however I am confused about the different scaling options and their impact on the meaning of the plot.
scale = covariance biplot (scale = 1), form biplot (scale = 0). When scale = 1, the inner product between the variables approximates the covariance and the distance between the points approximates the Mahalanobis distance.
How To Read Biplot
obs.scale = scale factor to apply to observations
How To Interpret Pca Biplot
var.scale = scale factor to apply to variables
I am not sure which of these scaling options I should choose for a prcomp object? I have seen lots of people set obs.scale = 1 and var.scale = 1, but I don't understand the reason for this.
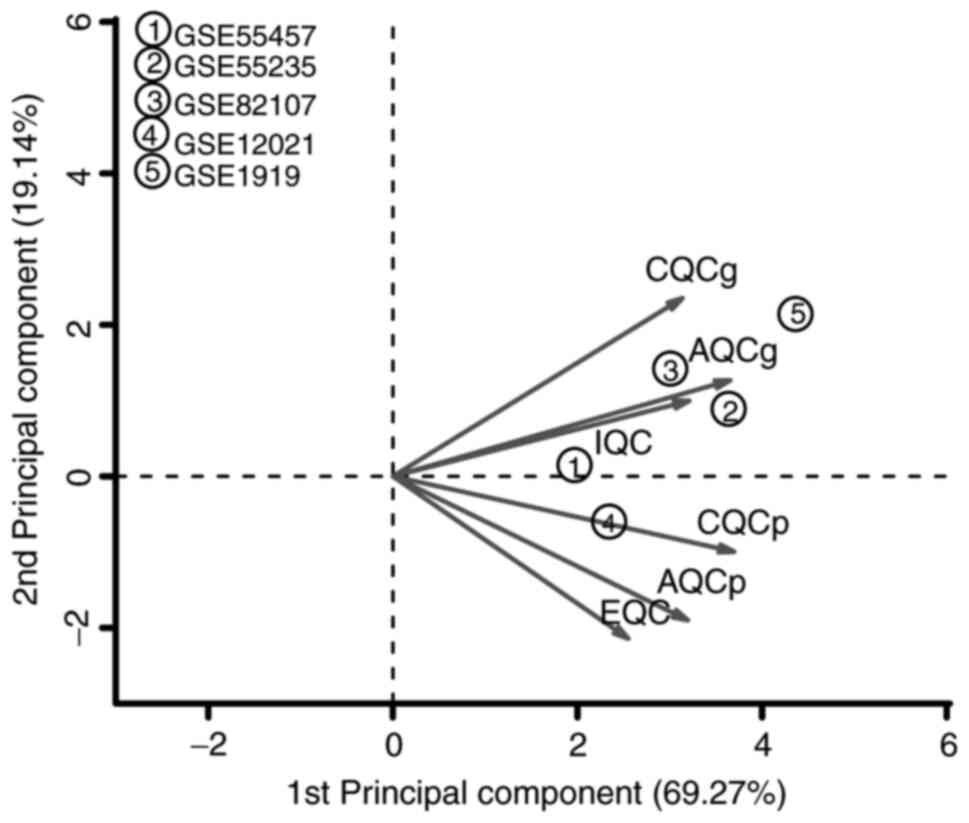
What does the line length and angle actually correspond to in a biplot? I get the general idea that a high value on PC1 indicates that the variable has a strong influence on PC1 whilst a small value indicates a small influence. And that if the arrow is pointing to the right, then that variable has a positive impact on the PC.

Thanks for the help!!
Best wishes,
Lucy